10.1 Wrangling Setup
20180908 Packages used in this chapter include dplyr (Wickham et al. 2023), FSelector (Romanski, Kotthoff, and Schratz 2023), ggplot2 (Wickham et al. 2024), glue (Hester and Bryan 2024), janitor (Firke 2023), lobstr (Wickham 2022), lubridate (Spinu, Grolemund, and Wickham 2023), randomForest (Breiman et al. 2022), readr (Wickham, Hester, and Bryan 2023), stringi (Gagolewski et al. 2023), stringr (Wickham 2023), tidyr (Wickham, Vaughan, and Girlich 2024), magrittr (Bache and Wickham 2022), and rattle (G. Williams 2023).
Packages are loaded into the currently running R session from your
local library directories on disk. Missing packages can be installed
using utils::install.packages() within R. On Ubuntu, for
example, R packages can also be installed using $ wajig install r-cran-<pkgname>
.
# Load required packages from local library into the R session.
library(rattle) # weather dataset.
library(readr) # Efficient reading of CSV data.
library(dplyr) # Wrangling: glimpse().
library(lobstr) # Inspect R data structures.
library(tidyr) # Prepare a tidy dataset, gather().
library(magrittr) # Pipes %>% and %T>% and equals().
library(glue) # Format strings.
library(janitor) # Cleanup: clean_names().
library(lubridate) # Dates and time.
library(FSelector) # Feature selection, information.gain().
library(stringi) # String concat operator %s+%.
library(stringr) # String operations.
library(randomForest) # Impute missing values with na.roughfix().
library(ggplot2) # Visualise data.
library(purrr) # simplify(), set_names()
The rattle::weatherAUS dataset is loaded into the template
variable ds
and further template variables are setup as
introduced by Graham J. Williams (2017). See
Chapter 8 for details.
<- "weatherAUS"
dsname <- get(dsname)
ds
<- nrow(ds)
nobs
<- names(ds)
vnames %<>% clean_names(numerals="right")
ds names(vnames) <- names(ds)
<- names(ds)
vars <- "rain_tomorrow"
target <- c(target, vars) %>% unique() %>% rev() vars
References
Your donation will support ongoing availability and give you access to the PDF version of this book. Desktop Survival Guides include Data Science, GNU/Linux, and MLHub. Books available on Amazon include Data Mining with Rattle and Essentials of Data Science. Popular open source software includes rattle, wajig, and mlhub. Hosted by Togaware, a pioneer of free and open source software since 1984. Copyright © 1995-2022 Graham.Williams@togaware.com Creative Commons Attribution-ShareAlike 4.0
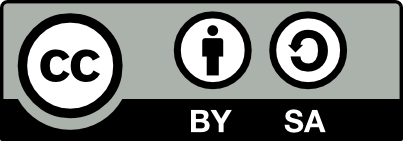