10.55 Variable Roles
20180723 Now that we have a basic idea of the size and shape and contents of the dataset and have performed some basic data type identification and conversion we are in a position to identify the roles played by the variables within the dataset. First we will record the list of available variables so that we might reference them below.
## [1] "date" "location" "min_temp" "max_temp"
## [5] "rainfall" "evaporation" "sunshine" "wind_gust_dir"
## [9] "wind_gust_speed" "wind_dir_9am" "wind_dir_3pm" "wind_speed_9am"
## [13] "wind_speed_3pm" "humidity_9am" "humidity_3pm" "pressure_9am"
## [17] "pressure_3pm" "cloud_9am" "cloud_3pm" "temp_9am"
## [21] "temp_3pm" "rain_today" "risk_mm" "rain_tomorrow"
By this stage of the project we will usually have identified a
business problem that is the focus of attention. In our case we will
assume it is to build a predictive analytics model to predict the
chance of it raining tomorrow given the observation of today’s
weather. In this case the variable rain_tomorrow
is the
target variable. Given today’s
observations of the weather this is what we want to predict. The
dataset we have is then a training dataset of historic observations. The task is to identify any
patterns among the other observed variables that suggest that it rains
the following day.
# Note the target variable.
target <- "rain_tomorrow"
# Place the target variable at the beginning of the vars.
vars <- c(target, vars) %>% unique() %T>% print()
## [1] "rain_tomorrow" "date" "location" "min_temp"
## [5] "max_temp" "rainfall" "evaporation" "sunshine"
## [9] "wind_gust_dir" "wind_gust_speed" "wind_dir_9am" "wind_dir_3pm"
## [13] "wind_speed_9am" "wind_speed_3pm" "humidity_9am" "humidity_3pm"
## [17] "pressure_9am" "pressure_3pm" "cloud_9am" "cloud_3pm"
## [21] "temp_9am" "temp_3pm" "rain_today" "risk_mm"
We have taken the opportunity here to move the target variable to be
the first in the vector of variables recorded in
vars
. This is common practice where the first variable in
a dataset is the target (dependent variable) and the remainder are the
variables (the independent variables) that will be used to build a
model to predict that target.
Your donation will support ongoing availability and give you access to the PDF version of this book. Desktop Survival Guides include Data Science, GNU/Linux, and MLHub. Books available on Amazon include Data Mining with Rattle and Essentials of Data Science. Popular open source software includes rattle, wajig, and mlhub. Hosted by Togaware, a pioneer of free and open source software since 1984. Copyright © 1995-2022 Graham.Williams@togaware.com Creative Commons Attribution-ShareAlike 4.0
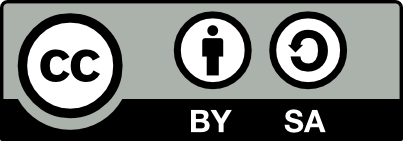