10.69 Identify Numeric and Categoric Variables
20180726 Identifying numeric and categoric variables may be useful for example for cluster analysis algorithms that only deal with numeric variables. Here we identify them by name (a character string) and by index. When using the index we have to assume the variables remain in the same order within the dataset and all variables are present, otherwise the indicies will get out of sync.
# Identify the numeric variables by index.
ds %>%
sapply(is.numeric) %>%
which() %>%
intersect(inputi) %T>%
print() ->
numi
## [1] 3 4 5 6 7 9 12 13 14 15 16 18 19
## [1] "min_temp" "max_temp" "rainfall" "evaporation"
## [5] "sunshine" "wind_gust_speed" "wind_speed_9am" "wind_speed_3pm"
## [9] "humidity_9am" "humidity_3pm" "pressure_9am" "cloud_9am"
## [13] "cloud_3pm"
# Identify the categoric variables by index and then name.
ds %>%
sapply(is.factor) %>%
which() %>%
intersect(inputi) %T>%
print() ->
cati
## [1] 8 10 11 22
## [1] "wind_gust_dir" "wind_dir_9am" "wind_dir_3pm" "rain_today"
Your donation will support ongoing availability and give you access to the PDF version of this book. Desktop Survival Guides include Data Science, GNU/Linux, and MLHub. Books available on Amazon include Data Mining with Rattle and Essentials of Data Science. Popular open source software includes rattle, wajig, and mlhub. Hosted by Togaware, a pioneer of free and open source software since 1984. Copyright © 1995-2022 Graham.Williams@togaware.com Creative Commons Attribution-ShareAlike 4.0
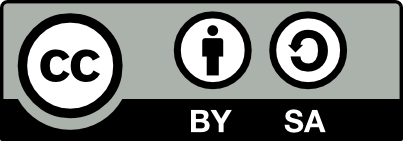