21.10 Conversion to Lower Case
General character processing functions in R can be used to transform our corpus. A common requirement is to map the documents to lower case, using base::tolower(). As above, we need to wrap such functions with a tm::content_transformer():
Your donation will support ongoing availability and give you access to the PDF version of this book. Desktop Survival Guides include Data Science, GNU/Linux, and MLHub. Books available on Amazon include Data Mining with Rattle and Essentials of Data Science. Popular open source software includes rattle, wajig, and mlhub. Hosted by Togaware, a pioneer of free and open source software since 1984. Copyright © 1995-2022 Graham.Williams@togaware.com Creative Commons Attribution-ShareAlike 4.0
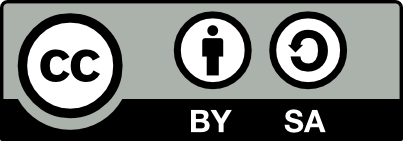