32 RelM Differential Privacy
20210327
This package demonstrates the utilisation of differential privacy to protect the privacy of data within a database. A query of the database will return the data perturbed by a certain amount. That is, if the actual result is 243, the query might return 245 one time, 242 another time.
The differential privacy libraries support the concept of a privacy budget which allows a certain number of queries before further queries can no longer assure privacy. Intuitively, the more perturbation, the larger is the privacy budget, and so the more queries that can be supported before the budget is spent.
The source code for this MLHub package is available from github: https://github.com/gjwgit/relm.
The use of differential privacy in health has been introduced in The application of differential privacy to health data and Practicing Differential Privacy in Health Care: A Review.
Your donation will support ongoing availability and give you access to the PDF version of this book. Desktop Survival Guides include Data Science, GNU/Linux, and MLHub. Books available on Amazon include Data Mining with Rattle and Essentials of Data Science. Popular open source software includes rattle, wajig, and mlhub. Hosted by Togaware, a pioneer of free and open source software since 1984. Copyright © 1995-2022 Graham.Williams@togaware.com Creative Commons Attribution-ShareAlike 4.0
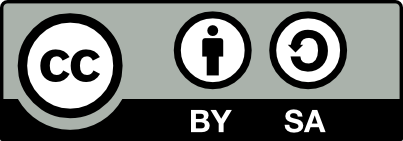