7.6 kmeans train
20220104
You can supply your own dataset as a csv file with a column for each variable, with at least one column. , a row for each instance, and at least your chosen K number of rows. The data is assumed to be numeric and to have been normalised (e.g, converted to numbers in the range -1 to 1) so that no variable dominates any other variable in calculating distances (a distance of 10,000 for incomes of $40,000 and $50,000 swamps a distance of 10 for ages of 40 and 50). See normalise for a helper function to normalise your data.
Common usage:
Typical output:
sepal_length,sepal_width,petal_length,petal_width,label
6.85,3.07,5.71,2.05,0
5.00,3.42,1.46,0.24,1
5.88,2.74,4.38,1.43,2
Note that the algorithm initialises itβs starting point (the first k centres) randomly, and so the model that is built each time may be quite different.
General usage:
$ ml train kmeans [OPTIONS] K [FILENAME]
-o <model.csv> --output=<model.csv> Filename of the CSV file to save model, or to STDOUT.
-m <movie.mpg> --movie=<movie.mpg> Filename of the movie file to save if desired.
-v --view Popup a movie viewer to visualise the algorithm.
--help Show this message and exit.
The output from train is also a csv file with k rows and a header row. Each of the k rows correspond to a βdiscoveredβ or βfitβ cluster. This is the trained model. The cluster is represented as the central point of the cluster, calculated as the βmeanβ or βaverageβ of each of the variables in the dataset across all the points/people in that cluster.
We can use these centroids to place (predict) new observations (people) into one of the k clusters.
The parameter k needs to be provided up frontβi.e., we need to guess a suitable value for the number of clusters. There are other tools that can assist in deciding on a good value for k.
With no csv specified on the command line the csv data (with a header row) is read from standard input. This allows the command to be part of a pipeline of commands, whereby the training data could be piped from another operation.
The default output written to standard output is a csv of the centres, with a cluster label appended to each row, and a header row with the cluster label column named label. Standard output can be redirected to a file or consumed through a pipeline.
If no csv output is specified then the output is always to the
terminal, irrespective of whether a mp4 is also output or whether
--view
is requested.
The output can be saved into a file simply by redirecting standard out (stdout):
Your donation will support ongoing availability and give you access to the PDF version of this book. Desktop Survival Guides include Data Science, GNU/Linux, and MLHub. Books available on Amazon include Data Mining with Rattle and Essentials of Data Science. Popular open source software includes rattle, wajig, and mlhub. Hosted by Togaware, a pioneer of free and open source software since 1984. Copyright © 1995-2022 Graham.Williams@togaware.com Creative Commons Attribution-ShareAlike 4.0
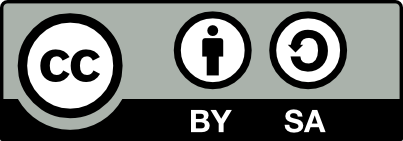